Clinical trials for rare diseases
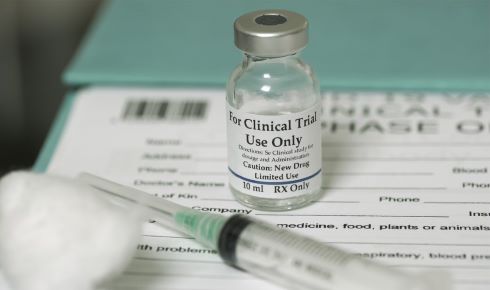
29th February 2024
Clinical trials traditionally test new treatments on large cohorts of patients. But what if the treatment is for a condition which affects a very small number of people?
In the UK, a disease is considered rare if it affects fewer than one person in 2,000. The low prevalence of these diseases often makes it challenging to conduct conventional randomised clinical trials.
It can be difficult to recruit rare disease patients for multiple reasons. “With rare diseases, individual patients are located in many different places and seen by different providers,” says Hilary Vernon, associate professor of genetic medicine at the Johns Hopkins University School of Medicine. “Without a well-defined natural history, it can be very difficult to select clinical trial endpoints.”
And often there are just not enough patients to begin with. For instance, Vernon’s research group investigates the Barth syndrome, a genetic disease that impairs lipid metabolism among males and is so ultra-rare that there are only 200-300 known cases in the world.
With trials for common diseases, you have a lot of patients in each trial, making it easy to statistically spot even a small treatment effect. On the contrary, rare disease trials often have few participants. “We would need to see a comparatively large treatment effect to compensate for the small number of patients who could participate in a clinical trial,” says Vernon.
And if the disease is very rare, Vernon adds, you may not be able to do a replication study. New approaches to clinical trial design and analysis are enabling a paradigm of small-n trials to serve the needs of rare disease patients.
Rethinking clinical trials
Electronic health records can improve recruitment in rare disease clinical trials. “If you look at electronic health records, you could find out where there may be more people living with the condition and try and recruit through those sites,” says Matthew Sydes, professor of clinical trials and methodology at University College London.
Sometimes, it might be prudent to work with a network of these sites. For example, osteosarcoma is a rare bone cancer with only about 150 to 200 cases a year in the UK. By working together in a network of 17 countries, Sydes and colleagues could deliver what was then the largest randomized controlled trial for osteosarcoma. It was a basket trial that investigated multiple chemotherapies at once.
In addition to such collaborations, adaptive trials allow researchers to make the most of limited patient populations. These are trials that permit modifications to the trial design based on accumulating evidence. This way, researchers can ascertain the efficacy of a drug with fewer patients, while ensuring that more of them receive the treatment dose as compared to traditional clinical trials.
Take multi-arm multi-stage (MAMS) trials, for instance. “These trials allow researchers to efficiently address several questions within one clinical trial protocol,” says Sydes. There are multiple treatment arms against a control arm and at each stage, assessment of some treatment arms can be stopped early, and others can be introduced. Some treatment arms may be dropped or added. “By systematically moving away from the things that aren't working well enough, you focus down on the ones that are looking interesting,” Sydes adds.
With sequential multiple assignment randomized trials (SMART), the patients are randomized to a sequence of treatments, with different decisions for those who respond and those who don’t at each stage. Small-n SMART (or snSMART) flip these trials on their head.
“We use multiple stages to gain more information from a small number of people,” says Kelley Kidwell, associate professor of Biostatistics at the University of Michigan School of Public Health.
Instead of different treatments, they can also be used for different dose levels. “You will be randomised to placebo, low and high dose. And if you're first randomised to placebo, you get re-randomised to low or high dose,” says Kidwell. This is a significant incentive as every patient in the trial would receive an active level of the dose, particularly since rare disease trials find it harder to recruit.
But that has its limitations. “Since you treat the same patient multiple times, that can potentially introduce bias in estimating the treatment effects,” says Tianjian Zhou, an assistant professor of statistics at Colorado State University. Moreover, they're only appropriate for chronic rare diseases that remain relatively stable throughout the trial. “It's also not relevant for all treatments if it's going to be a curative treatment,” adds Kidwell.
“For a lot of rare diseases, validated biomarkers have not been established,” says Vernon. “I think allowing for more generous criteria for validating a biomarker, or acceptance of less conventional biomarkers, could be extremely helpful.”
Borrowing information
In a traditional clinical trial, the treatment effect is estimated by data collected during a trial. This approach doesn’t always suit adaptive trials, small-n trials, and new lines of evidence for rare disease drug development. They are better served with Bayesian models, as compared to the frequentist nature of traditional trials. In Bayesian statistics, the treatment effect is not a fixed parameter to be estimated, but a degree of belief (that the treatment works) that is updated with every piece of evidence.
Some of this evidence is collected during the trial, such as the different stages of a MAMS trial or different treatments in a SMART. But this evidence can also come from before the trial, such as previous natural history data of the disease, previous clinical trials, or real-world data gathered from electronic health records or wearables.
Speaking on Bayesian methods, Zhou says that “they help us to bring in existing information or information that is outside the current clinical trial. This permits what is called borrowing of information. In other words, if the drug performs well in a common cancer type, it can provide some additional information for a rare cancer type. It could make the estimate more precise, or you don't need as many patients with the rare cancer types to get a precise estimate of the treatment effect.”
Often, the control is not a placebo but an existing drug that is the standard of care and benchmark for the new drugs under trial. Bayesian analyses allow utilizing existing information on the control drug to reduce the size of the control arm. “You can either enrol fewer patients on the control arm or you can completely ignore the control arm and perform a single-arm trial,” says Zhou.
In a single-arm trial, all participants receive the investigational drug. These trials look at a patient’s condition before and after a trial, compared to a baseline determined before the trial. Think of it as how a person goes through multiple shampoo brands before settling on one. These trials have been used effectively in developing therapies for rare cancers.
More recently, scientists have been integrating data from real-world sources to generate synthetic control arms. This is beneficial for ultrarare diseases but also rare diseases where a lower sample size is desired. For example, though many rare genetic diseases onset early in life, ethical considerations necessitate that few children be recruited to clinical trials. Bayesian approaches can be used to extrapolate insights from adults to children.
“Rare diseases can affect people quite differently,” says Kidwell. Subtypes in rare diseases complicate endpoints and often fragment the already small patient populations. “Bayesian methods might allow us to meet these global inference procedures and get more precision across multiple endpoints,” Kidwell adds. At the extreme of this paradigm are n-of-1 trials that look at how an individual patient responds to a drug. As of now, these are mainly limited to clinical care instead of developing new drugs.
Regulatory status
Regulatory bodies are warming up to some of these new approaches to expand rare disease clinical trials. “FDA’s Complex Innovative Designs initiative is looking for these types of clinical trial designs using Bayesian methods,” says Kidwell. The FDA is also open to accepting real-world evidence to support rare disease drug development. In the UK, the Medicines and Healthcare products Regulatory Agency (MHRA) is also developing guidelines for using real-world data as an external control arm.
FDA is also enabling single-arm trials beyond rare cancers. For example, it confirmed that an ongoing single-arm phase 1/2 trial would suffice for accelerated approval for a new therapeutic vaccine for recurrent respiratory papillomatosis, a rare infection. Other approaches are acceptable for some parts of clinical trials. Regulatory agencies are willing to let researchers use natural history data to help guide clinical trial design.
Most of the rare diseases that garner any attention are relatively more common or have strong patient groups to advocate for them. Increased collaboration between all stakeholders and wider regulatory acceptance of innovative clinical trial designs will change that, benefitting more rare disease patients.
Sachin Rawat is a freelance science and tech writer based in Bangalore. He holds a master’s degree in biotechnology.