AI versus the human brain
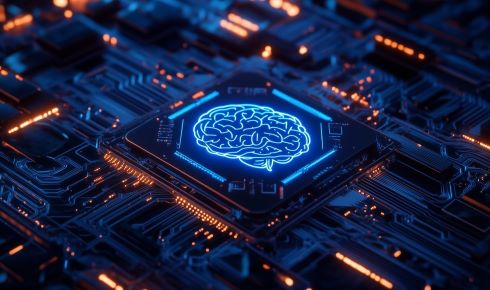
6th December 2024
AI expert James V Stone puts the hyperbole about the future of artificial intelligence in context by reminding us that AI systems are not anything like human brains – yet
Artificial intelligence (AI) researchers come in two broad varieties: scientists and technologists. The scientists attempt to build artificial general intelligence (AGI) systems – that is, systems capable of tackling a wide range of tasks, not just the specialist task they have been trained on. In other words, an AI that could think like a human. In contrast, technologists are not worried about whether a system thinks like a human. They just want a system that solves a difficult problem.
Modern AI systems make extensive use of artificial neural networks, which are computer models analogous to, but much simpler than, networks of biological neurons. Like biological neurons, artificial neural networks learn by adjusting the strengths of connections between neurons, but the similarity ends there.
The workhorse of AI systems is the ‘back-propagation algorithm’ (co-invented by 2024 Nobel Prize winner Geoffrey Hinton), which is a method for finding the correct connection strengths, but is generally acknowledged to be implausible as a biological learning mechanism. For an artificial neuron, the relationship between its inputs and its output state is usually pretty simple, typically taking the form of a semi-linear S-shaped activation function. More importantly, this output state does not depend in any way on previous inputs, so the neuron’s output has no memory of inputs received in the recent past. In contrast, a biological neuron is a more intricate system with complex dynamics: its ‘output’ consists of continuous changes in membrane voltage and in the rate at which action potentials are generated, and both of these depend on the inputs received in the recent past.
In terms of size, the human brain has 86 billion neurons, each of which has about 7,000 synaptic connections, making a total of around 600 trillion (6×1014) synapses. This suggests that the brain has about 300 times as many connections as ChatGPT-4. However, it is unlikely that the competence of these systems is purely a matter of size. A bee’s brain has only around 100,000 neurons, but it can still perform complex tasks¹.
As conscious as a brick
Because modern AI systems are based on artificial neural networks, it is tempting to believe that they operate like human brains. However, even a cursory comparison between AI systems and brains reveals fundamental differences. Of these differences, the most obvious concerns temporal dynamics: AI systems such as ChatGPT do not have any. When two humans are having a conversation, their brains do not come to a complete standstill when the conversation is over; they do not lapse into unconsciousness, to wake again only if someone speaks to them. In contrast, after a conversation is over, a ChatGPT system simply stops; there is no ongoing activity, as there always is for a brain. In other words, these systems have no internal dynamics. When they are receiving no inputs, they have more in common with a brick than a biological brain.
Conversation point
A large language model (LLM) such as ChatGPT might appear to be intelligent because it seems to understand the questions we ask. However, ChatGPT is essentially a sophisticated programme for predicting the next word in a sentence². Being able to predict the next word accurately many times over to form entire passages of text is impressive, but it cannot provide the problem-solving abilities demanded of an AGI.
For example, ChatGPT cannot play checkers and when it was asked (at the time of writing) if Bell’s theorem (a theorem in quantum physics) could be false, it produced a long answer, which began with “Yes, Bell’s theorem could be false”, when, in fact, a theorem is, by definition, true. When asked, it gave the correct definition of a theorem, but then repeated its earlier error about Bell’s theorem. Recent papers report that the basic reasoning abilities of LLMs are limited³, that they seem to get distracted if irrelevant information is added to a question, and that they often fail on tests of intuition or common sense⁴. Humans, by contrast, switch easily between intuitive and more deliberative thinking.
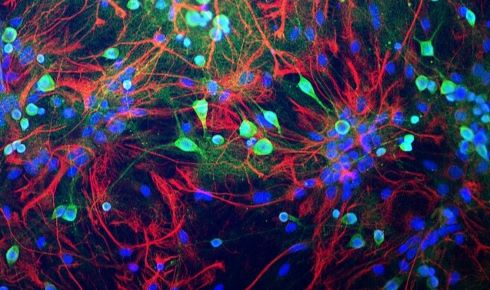
Yet because human lives revolve around language, and humans are the only species with sophisticated language, we are naturally (perhaps egotistically) impressed by a machine that seems to be linguistically skilled. However, as far back as 1966, simple language models such as ELIZA⁵ could interact with humans via a computer keyboard with responses that included phrases copied from previous parts of the conversation. Consequently, and despite its limited linguistic abilities, many users claimed that ELIZA appeared to possess a degree of empathy.
Given that the relatively simple ELIZA model fooled many humans into believing that it had empathy, we should not be surprised that ChatGPT is considered to be intelligent, or even sentient by some⁶. Claude Shannon, a prominent pioneer of AI, said: “I confidently expect that within 10 to 15 years we will find emerging from the laboratory something not too far from the robots of science-fiction fame⁷.” That was in 1961! This year, founder of DeepMind and 2024 Nobel Prize winner Demis Hassabis said that a general-purpose AI (AGI) appearing “within 10 years is a safe bet⁸”.
As demonstrated by Shannon and Hassabis (among others), this seems to be an infinitely renewable prediction. On the day an AGI system appears (if it ever does), whoever happened to repeat that prediction within the previous decade will be correct, but this is like predicting the next stock market crash – if the prediction is made often enough, then one day that prediction will inevitably be correct.
Strategic strength
Meanwhile, the technologists are not worried about how well their artificial neural networks mimic the human brain. What matters to them is whether their system can solve a single difficult problem. One active area of intelligence research is game-playing, but AI systems do not necessarily learn to play in the same way that humans do. When humans learn a game such as Go, they learn from other humans. In contrast, when an AI learns to play Go, it can do so by playing a clone of itself, so it has to create its own strategies. Consequently, some of the strategies adopted by AI are very different to those used by humans.
When DeepMind’s AlphaGo-Zero⁹ AI system beat one of the world’s best Go players in 2016¹⁰, it did so with a move that was so outrageous, and so original, that the experts observing the game initially thought the AI system had made a serious error. In fact, the strategies created by AI systems are so novel that they have changed the way that games such as Go and chess are played by world-class human players. It turns out that not having a human teacher can provide substantial advantages for developing new strategies.
Somewhat surprisingly, the LLM architecture used in AI systems such as ChatGPT has been adapted for use in biotech applications using biomolecular units rather than words. One of the most important breakthroughs has been DeepMind’s AlphaFold¹¹, for which its inventors received the 2024 Nobel Prize in Chemistry. This system has made enormous progress on the long-standing problem of predicting the three-dimensional structure of a protein from its amino acid sequence.
Before the advent of AlphaFold, solving a single protein structure could take months or even years, and the number of proteins solved before AlphaFold existed was measured in the hundreds of thousands. AlphaFold has now predicted the three-dimensional structure of almost every one of the 200 million proteins in the UniProt database.
Recently, researchers at Google DeepMind introduced AlphaProteo¹², an AI system for designing novel, high-strength protein binders, which they claim can achieve better binding affinities than existing methods. The LLM architecture has also been used in so-called foundational models, such as the Evo model¹³. This general-purpose, seven-billion-parameter model was trained using whole-genome nucleotide sequences, so it can help to design new genome-scale sequences and predict a measure of the biological fitness of the protein sequences encoded by specific nucleotide sequences. In drug discovery, an AI system can rapidly screen millions of drugs to find those that bind to particular biological receptors¹⁴. As seen throughout our special issue of The Biologist, AI is driving powerful new understanding and innovations across the whole of the life sciences.
Yet despite the impressive achievements of these specialist AI systems, Shannon’s general-purpose science-fiction robot is still patiently waiting for us. And it is still about 10 years in our future, where it may remain for many decades to come.
James V Stone is a visiting professor at the University of Sheffield and author of The Artificial Intelligence Papers: Original Research Papers with Tutorial Commentaries
1) Bridges, A. et al. Bumblebees socially learn behaviour too complex to innovate alone. Nature 627, 572–578 (2024).
2) For a superb video tutorial, see How Large Language Models Work: A Visual Intro to Transformers. Chapter 5: Deep Learning.
3) Mirzadeh, I. et al. GSM-Symbolic: Understanding the limitations of mathematical reasoning in large language models. (October 2024).
4) Kejriwal, M. et al. To find out how smart AI is, test its common sense. Nature 634, 291–294 (2024).
5) Weizenbaum, J. ELIZA – A computer program for the study of natural language communication between man and machine. Comm. ACM 9(1), 36–45 (1966).
6) De Cosmo, L. Google engineer claims AI chatbot is sentient. Sci. Am. 12 July 2022.
7) ‘The Thinking Machine’ (1961) with Claude Shannon from the Tomorrow documentary series.
8) Google DeepMind: The podcast with Demis Hassabis.
9) Silver, D. et al. Mastering the game of Go without human knowledge. Nature 550(7676), 354–359 (2024).
10) AlphaGo – The Movie (2020)
11) Jumper, J. et al. Highly accurate protein structure prediction with AlphaFold. Nature 596(7873), 583–589 (2021).
12) Zambaldi, V. et al. De novo design of high-affinity protein binders with AlphaProteo. Google DeepMind (2024).
13) Nguyen, E. et al. Sequence modelling and design from molecular to genome scale with Evo. BioRxiv, 2024–2002 (2024).
14) Blanco-Gonzalez, A. et al. The role of AI in drug discovery: Challenges, opportunities, and strategies. Pharmaceuticals 16(6), 891 (2023).
Acknowledgement Thanks to Raymond Lister for valuable feedback on this article.